Key Factors for Effective Online Chat
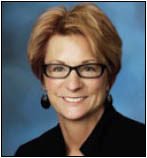
Online chat continues to develop as an influential, profitable customer service and sales delivery method. However, even with growing consumer preference, impressive ROI and high customer satisfaction, online chat remains an undeveloped channel. Forrester Research has predicted U.S. Online retail sales will reach nearly $250 billion by 2014. Increased consumer demand for web-based customer support and online shopping creates a tremendous opportunity for those companies that launch online chat effectively to capitalize on this demand.
Still a Limited Channel, But with Huge Benefits
Online chat delivers fast, personalized and timely communications to a customer. The benefits of a robust online chat presence are significant. Chat can reduce overall contact center costs by limiting average interaction costs. It can shorten the sales process by resolving customer issues immediately and it can help customers complete order forms before they abandon a transaction. It can increase transaction values with upsell and cross-sell opportunities while the customer is shopping online. Online chat also allows for increased efficiency as agents are able to multitask, handling multiple chats simultaneously while customers type in their questions or view information.
Despite these benefits, and the overall preference for online chat among many younger customers, chat is often unavailable, especially to support sales. When it is offered, the customer experience can be improved. With more consumers moving their business and support issues online, there is a tremendous opportunity for companies with a web presence to provide topnotch customer service and sales support by introducing a human element into the online interaction. The very best chat providers in all industries treat online customer service as a new frontier that demands sophisticated and specialized training for their agents. When launching a new chat queue in the contact center, there are several key tactics for optimizing the customer experience.
Optimize Agent Skills by Training for Chat
Training for online chat is one of the most important things a company can do to optimize the online customer experience. A good telesales agent is not necessarily a good chat sales agent. It is important to educate agents on the differences between chat and other forms of communication. Good managers will ensure that their chat agents understand how to construct a conversation flow that fits the needs of the customer and the company.
Best practices show that conversations should include multiple exchanges with back-and-forth messages. Many companies want to apply the 80/20 rule when it comes to canned versus freeform content. That means 80% of the most-asked questions have precrafted, hot-key responses that are grammatically correct and accurately reflect the company brand. Agent skills and training make up the other 20% providing the personalized, human interaction that is so critical to the overall customer experience. No matter what the ratio, it is important that all agents are aware of the target they should be aiming for as well as the company’s general guidelines to ensure consistency in responses.
The best chat agents should also be trained to consistently use problem-solving skills to troubleshoot online customer concerns. If required, the best agents should be able to transition the customer to Tier 2 agents without the customer ever being aware of the escalation. Higher-performing agents also tend to resolve problems online in the chat channel, but also provide the 800 number for later follow up.
The goal is to train chat agents to avoid some of the worst online chat mistakes including answering questions with other questions, providing inaccurate or conflicting information, sharing hyperlinks that don’t work and using unnecessary promotional copy. All of these require customer effort—making the customer work to figure out the answer in what should be a convenient and quick online support channel.
Invest in Writing Skills
Agents, particularly offshore, require training on basic writing skills to ensure a consistent, positive customer experience. Responses must adhere to most grammatical rules, including capitalization and punctuation. Accurate spelling is critical to support agent credibility.
Because chat users expect instant gratification, sentences need to be kept short and to the point. When structuring answers, managers should ensure that their agents are putting the most important information up front. While formal responses require the use of complete sentences, less formal responses may contain sentence fragments without sacrificing credibility. Further, while smiley faces and emoticons are often acceptable in a chat interaction, too many can appear unprofessional and detract from your company’s cultivated brand.
To effectively gauge the strength of your agent’s writing skills, managers should consider including typing tutorials to measure speed and accuracy of agent responses. In the end, writing effective chat communications can be an art and requires the right skill set coupled with dedicated chat training.
Understand How Communications Style Impacts Brand
In addition to writing skills, overall communications style is important. Voice and tone can be relatively easy to read on a telephone, but in an online chat session, they can be difficult to convey. When it comes to tone, chat agents need to ensure that their tone always reflects the corporate identity and conforms to the personality of the site (e.g., informative, professional, innovative). For voice, chat agents should try to humanize the online experience by ensuring that their interactions express the appropriate mood or feeling of the conversation (e.g. friendly, upbeat, conversational).
When it comes to communications style, agents need to make sure the language they use has mass U.S. appeal. Regional slang and clichés should be avoided, and the use of technical terms and acronyms should be minimized. Agents should, however, still aim to personalize their correspondence. For a best-in-class chat experience, agents should use the customer’s name and other customer-provided information as much as possible to strengthen the online connection with their customers.
Staff for Chat to Avoid Customer Frustration
Customers expect immediate real-time response from today’s online support channels. Significant lag times or dropped chats are not acceptable. Ultimately, customers should never have to ask: ”Are you still there?” This can lead to significant customer frustration and disengagement.
Many companies find synergies between email and chat support queues as both are writing oriented. Some companies can staff and crossskill agents effectively. However, keep in mind that normal email turnaround ranges between four to 24 hours, whereas the time for a best-in-class chat interaction is around 30 seconds. Therefore, staffing for chat to deliver brand-friendly, responsive customer service is critical. Other factors to consider when staffing for chat include whether you plan to offer proactive or reactive chat (or both), your posted days/hours of operations and the complexity of your products.
Ensure Consistent, Updated Product Knowledge
Integrating chat into the contact center is an important consideration that requires a holistic view. Ideally, all agents regardless of channel (voice, email, chat) will have access to a knowledge base that is meticulously maintained and updated with the latest product information as well as details on special offers and promotions to customers. Agents can quickly erode trust if they provide conflicting or inaccurate information.
Integration into the contact center CRM is also important. Empowered chat agents need to be able to access customer information and previous interaction histories. The chat platform should also support real-time collaboration and internal instant messaging among agents, supervisors and subject-matter experts within the contact center.
Know the Chat System Features
It is important that agents not only have access to the appropriate knowledge base, but are also fully trained on the chat technology platform itself and fully understand its functionality. Using the system to correctly set customer expectations is important. The best chat systems automatically update customers on their chat queue position and estimated wait time before reaching an agent. Once engaged, the chat system notifies the online chatter when the agent is typing a response. The ability to increase font sizes can also be an important feature as is the ability to obtain post-chat transcripts.
To fully maximize a customer’s experience, agents also need to understand the more advanced chat-related features. This includes co-browsing, document sharing and page pushing. Consider a website visitor on a shopping cart page. After some predetermined criteria, you invite the visitor to engage in a chat session for assistance in completing the order. Rather than finalize the transaction, however, the visitor now wants to compare other products. Through the power of the chat platform, and with the visitor’s permission, agents can then co-browse the website with the visitor or push web pages for further information. By understanding the powerful chat features available, agents have the ability to maximize each customer interaction.
Finally, it is important that agents have a clear understanding of the security and encryption characteristics of the chat system. When supplying credit card information online, many customers are incredibly sensitive about security. Providing upfront hyperlinks to security policies along with encrypted number masks for entering personal information in the chat window can put customers at ease during the online transaction.
Measure the Right Metrics
The metrics used to measure chat must promote a balance between efficiency and quality. Traditional metrics like average response time or number of chats per hour need to be combined with metrics the chat agent can control. This includes measuring the accuracy of responses, the quality of responses, the resolution rates and overall customer satisfaction (often measured by post-contact email surveys). Assessing agent chat transcripts is the easiest way to uncover problems and make chat more effective.
For sales chat programs, margin per chat is also a key metric to fully understand the ROI of the channel and potentially compare it to margin per call to determine cost advantages. Chat sales close rates would be an additional key metric that could be compared across voice and email channels to fully understand the benefits of an effective chat program.
In the end, there is tremendous opportunity for companies to offer online chat as a standard customer service tool and sales channel. The key is to launch online chat effectively to avoid customer frustration and potential brand damage. This involves a solid plan focused on agent skills, chat communications, proper staffing, chat system features and measuring success. A focus on these key areas will enhance the total customer chat experience.
Emily Millar is Vice President of Client Relations for Telus International, a global provider of BPO and contact center solutions.
– Reprinted with permission from Contact Center Pipeline, www.contactcenterpipeline.com