Harness The Power Of NLP And Machine Learning
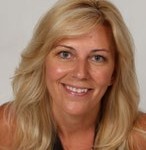
IT IS ESTIMATED THAT THERE ARE UP TO 7,000 LANGUAGES SPOKEN AROUND THE WORLD. We start as infants, learning sounds and patterns, growing to recognize rhythms and grammatical structures. Parents, teachers and others in our life help us in this journey, bringing us to a point where we can understand and communicate with confidence. With the rise of technology, computers have also embarked on this journey. While computers interpret things from a very literal point of view, they, like humans, must be taught how to decipher and understand human language.
Machine learning has its roots in statistical techniques and has evolved from pattern recognition and computational learning theory. The subject tends to involve concrete types of problems, involving large amounts of data. The intent of machine learning is to teach computers to learn patterns, predict behaviors and interpret data. To do this, the machine is shown labeled or partially labeled training examples, and learns the underlying patterns, allowing it to predict and interpret future examples.
Machine learning has been gaining traction and attention lately for its use in image recognition, speech-to-text and recommendation engines like the one used by Amazon, just to name a few applications. If you look closely, you’ll find that machine learning is an integral part of our daily lives.
Natural language processing (NLP) is an all-encompassing term for any kind of human language processing task, typically requiring both linguistic knowledge and machine learning foundations and techniques. Examples of NLP include speech recognition, language modeling, syntactic and semantic parsing, text classification, natural language generation and information retrieval (IR), to name a few. SIRI, Apple’s Personal Assistant, IBM Watson, the computer that won Jeopardy, and Google Translate, which uses statistical machine translation, are all notable examples of applied NLP with machine learning. These applications add a level of convenience to our lives by simplifying complex tasks, saving us a great deal of time and energy. Imagine how long it would take you to translate a document from English to French, while machine translation systems can perform this task nearly instantly.
How NLP Can Create Meaningful Customer Engagements
With the sheer amount of data being produced every day, it is thought that machine learning will play a critical role in the interpretation and consumption of this content. I recently had the opportunity to sit down with Daniel Pressel, chief science officer at Digital Roots, to understand how NLP is critical to creating meaningful digital, customer engagements. “What’s interesting is that people are better at producing data than they are at consuming it,” says Pressel. “The big question is how to unlock that content, to gain a better understanding of the problems at hand.”
As humans, we are pragmatic problem-solvers, seeking out straightforward solutions to our problems. We might imagine that a facial recognition tool must be powered by a very complex solution, but this perception doesn’t necessarily translate into problems that we have some experience with. The Internet is a massive body of data, but through the use of a search engine and a few keywords, we often can find a useful site very quickly. In fact, we have been conditioned to Google everything, typically making use of only the first few results. While this is enormously powerful for searching for a website for an answer, it quickly becomes intractable for understanding the many ways in which consumers describe their problems, interests, and experiences.
“We want to make sure that all customers are getting our attention. If they’re having problems, we want to be able to find and reach out to them no matter what language they speak or how they talk about a problem,” says Pressel.
A common limitation that Pressel sees in the space today is that the platform user is expected to be a data scientist, linguist and social media expert, all in addition to providing great customer service. In other words, the user must become an expert at manipulating multiple streams of social media and web content, searching for all of the different ways a person might phrase a problem or interest, while sifting the results down to only those that are relevant. Fine-tuning this equation manually is a monumental task, requiring constant tweaks. “A lot of times a search-based approach is just scratching the surface. As a field, customer service provides huge opportunity for expanding the use of NLP and machine learning, to overcome these limitations,” Pressel says.
People are better at producing data than consuming it. The big question is how to unlock that content, to gain a better understanding of the problems at hand.
Tools Combine NLP and Machine Learning Techniques
In his work, Pressel focuses on convenience and efficiency for users. Using NLP and machine learning techniques, tools are designed to automatically learn to recognize engagement opportunities, regardless of how they are expressed. Using such state-of- the-art techniques, users are able to find and assist customers at an unprecedented scale and with unparalleled precision. Additionally, the results are not static, as the machine can learn from user behavior, and can be taught to adapt to future needs by recognizing new data.
“A lot of what we do breaks down the customer experience. Understanding what the experience is for the customer, understanding if they’re having problems with the product, and especially knowing if this problem can be resolved,” says Pressel. Understanding how to solve customer problems is an important aspect, but Pressel also focuses on other challenges, such as understanding how customers feel about products, what they respond to, and how this information can be used to make better products and improve business processes.
While we may not think about them often, NLP and machine learning can greatly impact the experience for both the user (e.g., agents in the contact center) and, ultimately, the consumer that the agents are trying to serve. These techniques allow us to isolate issues, gain deeper understanding of products and services, and visualize emerging content as it’s happening. “These technologies mean happier consumers and happier agents,” says Pressel. “We’re reaching more customers by solving the problem directly—by teaching the computer what should be engaged and why.”
What Does the Future Hold?
In the next five to 10 years, Pressel predicts that there will be exciting changes in the space of NLP and machine learning. The recent explosion of data is exciting but challenging to navigate, and Pressel anticipates that, over time, we will get much better at understanding and using this data. New techniques utilizing not only supervised data, but also semi- and unsupervised data, will lead to improvements in system performance. At the same time, approaches are evolving to process data faster. Faster, deeper systems covering more data, with greater accuracy, are things that Pressel looks forward to in the near future.
“In the next five to 10 years, social analytics will look very different,” Pressel says. “I think people are going to realize that many of the techniques that are used today are not really optimal. We will be seeing systems that are smarter, specifically for call centers, systems that will help the agent handle more engagements, and do so more effectively.”
– Reprinted with permission from Contact Center Pipeline, http://www.contactcenterpipeline.com